Revolutionary MT-Net Model Boosts Lung Nodule Detection with 91.9% Accuracy Using EfficientUNetViT
September 23, 2024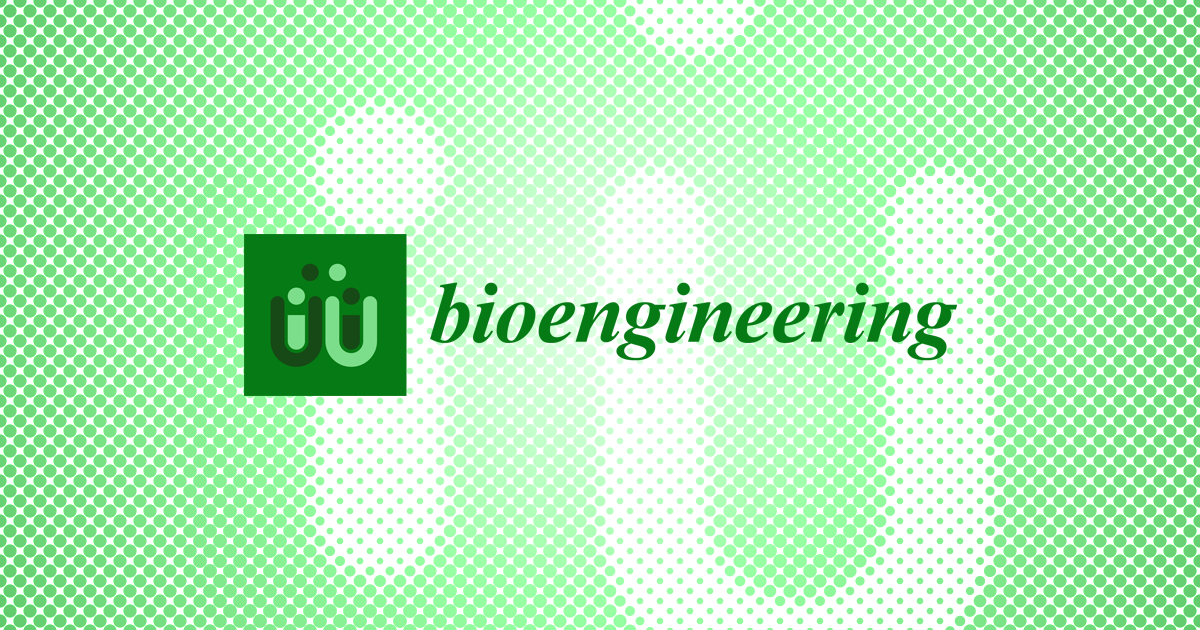
A new multi-task network, known as MT-Net, has been developed to simultaneously perform segmentation and classification of pulmonary nodules.
The MT-Net model utilizes the EfficientUNetViT architecture, which combines the global context capabilities of Vision Transformers (ViT) with the precise localization strengths of UNet for effective tumor segmentation.
Experimental results using the LIDC-IDRI dataset demonstrate that MT-Net achieves a Dice similarity coefficient of 83.2% for segmentation and an impressive accuracy of 91.9% for classification.
This innovative model shows competitive performance when compared to existing state-of-the-art methods in the field.
MT-Net is structured with three subnetworks: Coarse Seg-net for initial segmentation, Fine Seg-net for detailed segmentation, and Class-net for classification.
To enhance segmentation accuracy, MT-Net employs a hybrid loss function that combines Dice loss and rank loss, particularly improving performance at nodule boundaries.
The study also employs ANOVA analysis to assess the impact of various factors, including dataset characteristics and preprocessing techniques, on segmentation accuracy.
Findings indicate that a higher number of training epochs correlates positively with improved segmentation accuracy, highlighting the importance of sufficient training duration.
With pulmonary nodules detected in approximately 30% of chest CT scans, and the risk of malignancy increasing with nodule size, effective segmentation techniques are crucial for early diagnosis.
Lung cancer remains the leading cause of cancer-related deaths, underscoring the urgent need for advanced early diagnosis methods to improve patient outcomes.
Computer-aided diagnosis (CAD) systems are essential for enhancing diagnostic accuracy in lung cancer detection, which is critical given its high mortality rates.
The study emphasizes the superiority of deep learning techniques over traditional segmentation methods, showcasing their effectiveness in medical image analysis.
Summary based on 5 sources