Advancements and Challenges in AI: The Future of Large Language Models and Their Reasoning Abilities
October 20, 2024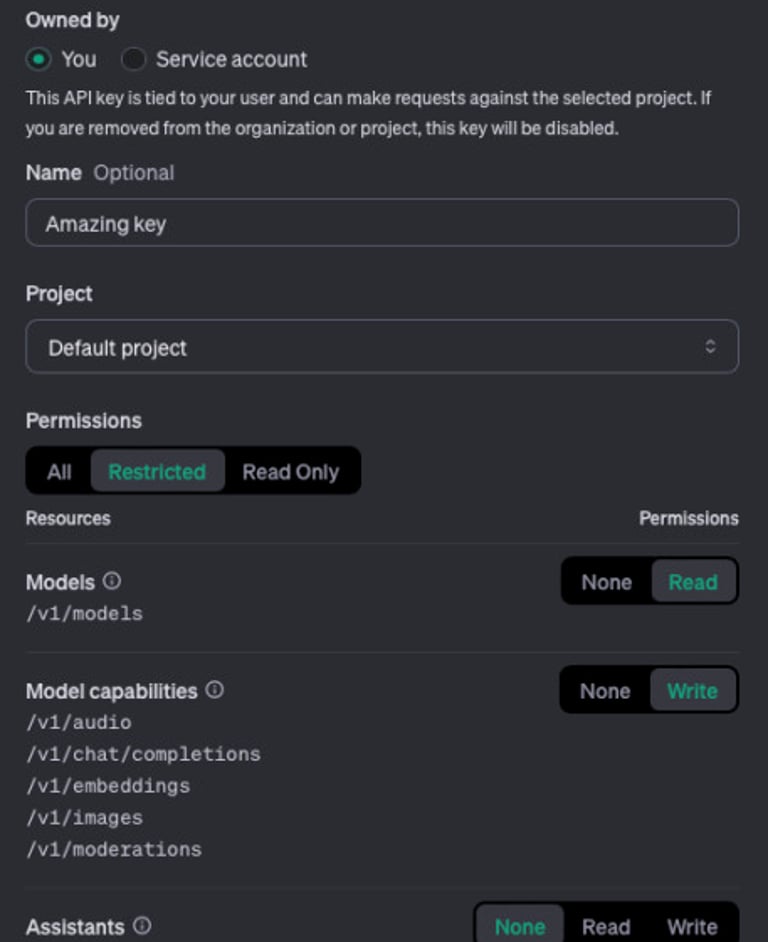
A forthcoming study titled 'What Are the Odds? Language Models Are Capable of Probabilistic Reasoning' will be presented at the 2024 EMNLP conference, focusing on evaluating and improving LLMs' reasoning abilities.
Eric Bravick, CEO of The Lifted Initiative, acknowledged the current limitations of LLMs but suggested that combining them with specialized AI subsystems could enhance accuracy in mathematical tasks.
While LLMs excel in text understanding and generation, they face challenges with numerical reasoning tasks, such as calculating probabilities.
These difficulties in numerical reasoning may stem from the training methods and the limited inclusion of numerical tasks in the training data.
Research indicates that LLMs have shown improved accuracy in diagnostic tasks, with performance on the MedQA dataset increasing significantly.
The importance of explainable AI is emphasized in building trust in LLMs and promoting their safe adoption in critical applications.
The review highlights significant gaps in assessing bias, fairness, and various healthcare tasks related to LLMs, underscoring the need for improved evaluation frameworks.
Overall, the implications of recent studies suggest a pressing need for enhanced reasoning capabilities and contextual understanding in future AI research.
The rise of large language models (LLMs) has significantly enhanced the capabilities of AI agents, enabling them to engage in natural, human-like conversations.
Challenges in fine-tuning LLMs include prompt refinement and the need for efficient strategies to enhance their capabilities.
These models allow AI agents to generate coherent ideas and conversations, integrating various modalities to achieve real-time coherence.
An effective approach to training LLMs involves making minimal modifications, focusing solely on key knowledge tokens to optimize performance.
Summary based on 17 sources
Get a daily email with more Tech stories
Sources
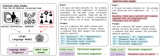
Nature • Oct 21, 2024
Evaluation and mitigation of cognitive biases in medical language models