WHO: 2 Billion Seniors by 2050; New AI Tech Tackles Fall Risks with WiFi and Video
October 4, 2024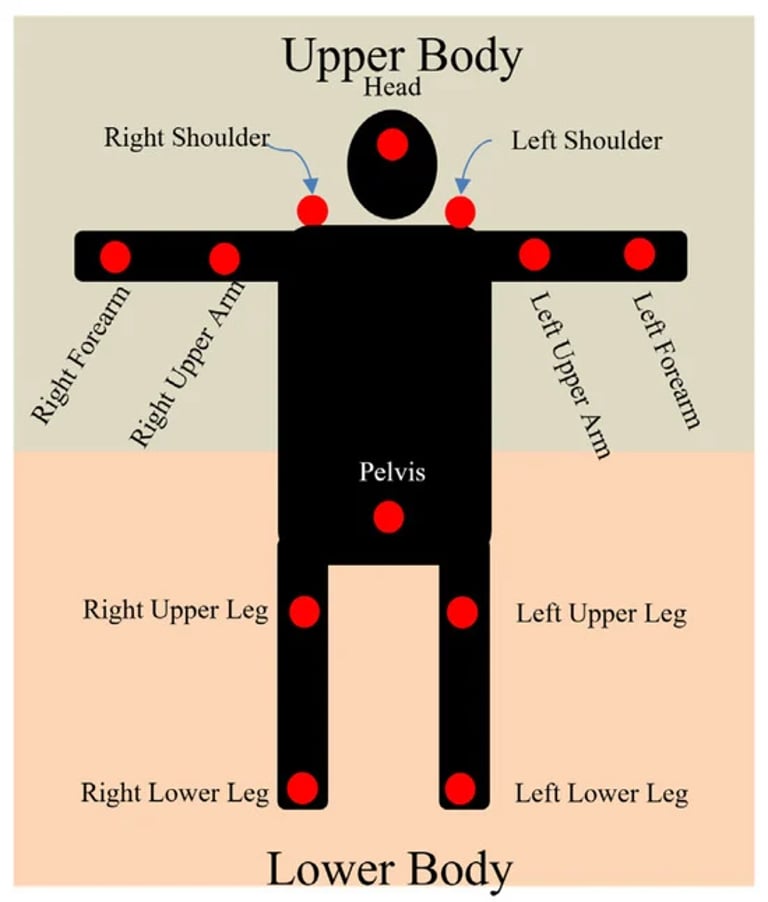
As the global population ages, the World Health Organization predicts that by 2050, there will be 2 billion people aged 60 and above, highlighting the urgent need for effective fall detection solutions.
Falls are a significant health risk for older adults, with an estimated 684,000 deaths annually attributed to falls, primarily affecting those over 60, and a yearly incidence of 28-35% for individuals over 65.
In this study, a cohort of 106 older adults performed the TUG test while wearing IMUs, with data collected at a sampling frequency of 100 Hz to ensure accuracy.
The findings support the integration of advanced machine learning techniques in clinical settings for more accurate and timely fall risk assessments in older adults.
An improved dual model for fall detection has been introduced, utilizing both video and WiFi signals to assess the health status of the elderly.
Video-based detection employs cameras and computer vision algorithms to analyze movements and identify falls, enhancing the accuracy of detection systems.
Recent research focuses on developing predictive models for assessing fall risk in older adults using data from wearable inertial measurement units (IMUs) during the Timed Up and Go (TUG) test.
The economic burden of falls is substantial, with medical costs in the U.S. attributed to falls estimated at approximately $50 billion annually.
WiFi signal-based detection uses Channel State Information (CSI) to analyze signal patterns for fall detection, providing a non-invasive alternative.
Support vector machine (SVM) classifiers have shown superior performance over neural networks in fall detection accuracy, demonstrating the effectiveness of machine learning in this field.
The proposed DAGAF decomposition method enhances feature extraction from accelerometer signals and holds promise for improving wearable fall detection systems.
This study also focuses on classifying fall directions—forward, backward, and sideways—alongside detecting non-fall activities like sitting and walking, which is crucial for tailoring medical interventions.
Summary based on 4 sources