Revolutionizing Oral Cancer Detection: Non-Invasive Diagnostic Tools Show Promise with 97.78% Accuracy
September 13, 2024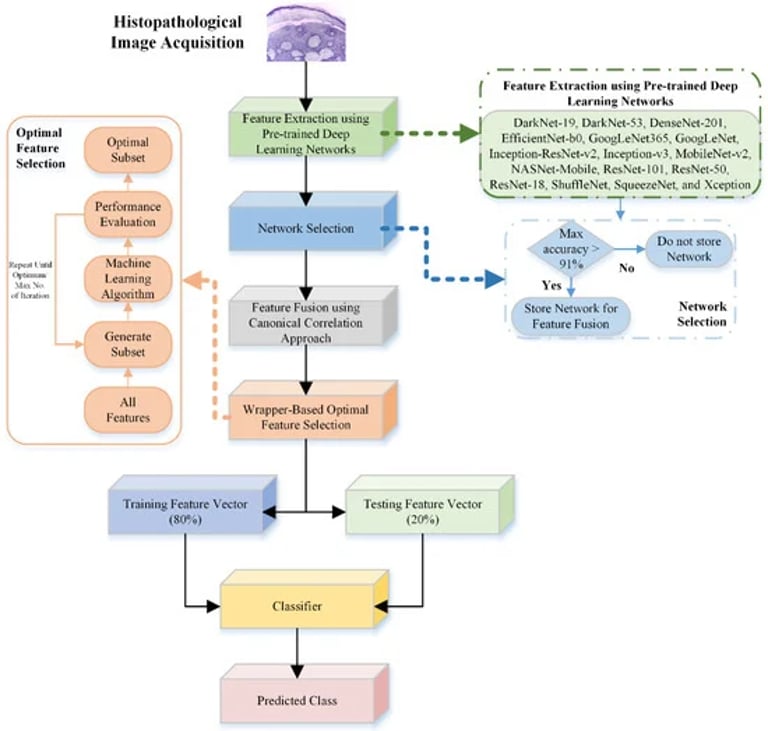
Light-based technologies, including chemiluminescence and autofluorescence, analyze differences in light absorption between healthy and abnormal tissues to assist in diagnosis.
The proposed method showed statistically significant results compared to other feature selection approaches, indicating its potential for clinical application.
Oral cancer, particularly oral squamous cell carcinoma (OSCC), is a major public health concern, with approximately 600,000 new cases and 300,000 deaths reported globally each year.
In 2020 alone, OSCC was responsible for 177,757 deaths, underscoring the critical need for early detection and effective diagnostic methods.
A recent systematic review focused on analyzing non-invasive methods for the early detection of OSCC and oral potentially malignant disorders (OPMDs), highlighting advancements in diagnostic technology.
Non-invasive diagnostic tools are emerging as promising alternatives, offering benefits such as reduced patient discomfort, objectivity, and cost-effectiveness.
Oral Brush Cytology is a minimally invasive method that collects exfoliated cells from lesions, aiding in the assessment of potential malignancies.
This automated detection framework could significantly enhance diagnostic efficiency and accuracy, ultimately improving patient prognosis.
However, despite these advancements, the review calls for further research to enhance the reliability of non-invasive diagnostic techniques for oral cancer screening.
The review identified four categories of non-invasive diagnostic tools: Vital Staining, Oral Brush Cytology, Light-Based Technology, and Spectroscopy.
Spectroscopy methods provide real-time diagnostics by detecting biochemical changes in tissues associated with cancer, demonstrating high sensitivity.
The study evaluates the effectiveness of various interpretable machine learning models in diagnosing leukoplakia and OSCC, proposing an automatic machine learning approach that achieved a classification rate of 97.78%.
Summary based on 3 sources