AI Breakthrough: Metabolomic Aging Clocks Predict Biological Age with Unmatched Accuracy
January 2, 2025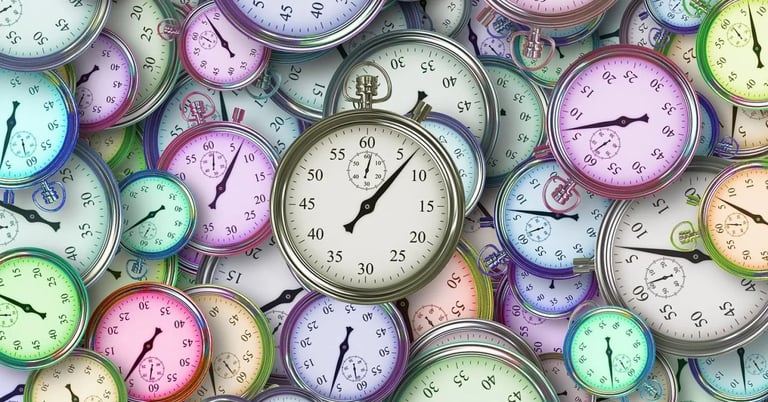
The research analyzed data from over 225,000 participants aged between 37 and 73 years, focusing on 168 metabolites in their blood plasma.
The analysis confirmed non-linear relationships between metabolites and age, suggesting the need for statistical corrections to enhance prediction accuracy.
Researchers at King's College London have published a groundbreaking study in Science Advances that explores the use of machine learning models to develop metabolomic aging clocks based on plasma metabolite data from the U.K. Biobank.
The study evaluated various AI algorithms to identify the most effective method for predicting biological age from human blood samples.
By comparing machine learning algorithms, the researchers aimed to enhance the accuracy of metabolomic aging clocks using nuclear magnetic resonance (NMR) spectroscopy data.
Among the algorithms tested, the Cubist regression model emerged as the most accurate, achieving a mean absolute error of just 5.31 years in predicting biological age.
The study introduced the concept of 'MileAge', which reflects the difference between predicted biological age and chronological age, with significant gaps indicating accelerated aging.
Notably, individuals with accelerated aging exhibited poorer health outcomes, including chronic illnesses and a frailty index score disparity of up to 18 years.
The findings revealed that a 1-year increase in MileAge delta was associated with a 4% rise in all-cause mortality risk, highlighting the serious implications of accelerated aging.
The researchers concluded that metabolomic aging clocks could serve as effective predictors of biological aging and health outcomes, paving the way for future clinical applications.
This research underscores the potential of aging clocks as biomarkers that could be tailored for studies on lifespan and health span extension.
Looking ahead, future research may focus on developing aging clocks based on tissues and cells to further deepen our understanding of the aging process.
Summary based on 2 sources
Get a daily email with more AI stories
Sources
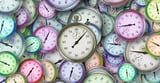
Psychology Today • Dec 31, 2024
Using AI to Predict Health and Longevity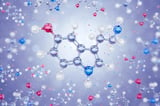
News-Medical • Jan 2, 2025
Machine learning reveals how metabolite profiles predict aging and health