Revolutionary YOLOv8 Algorithm Slashes Model Size, Boosts Accuracy for Real-Time Hazard Detection
September 12, 2024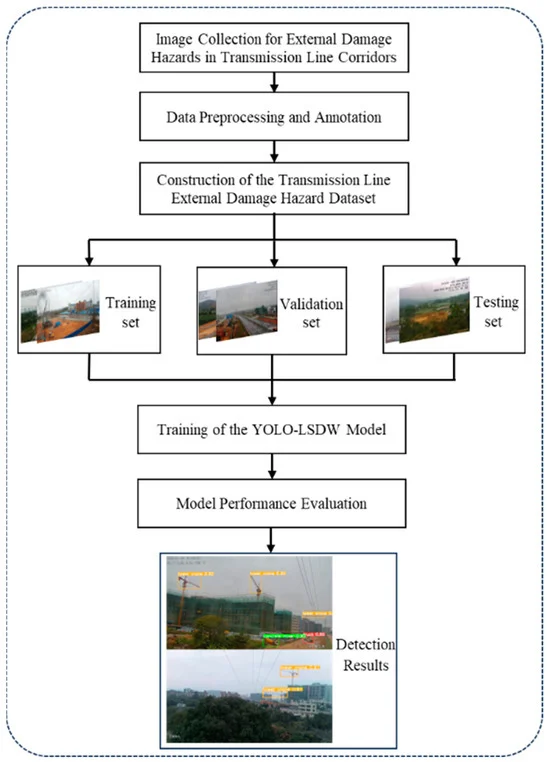
The YOLOv8 model architecture has been optimized for improved detection accuracy, employing advanced loss functions and a unified training framework, resulting in faster convergence and higher detection accuracy compared to the original model.
The GSL-YOLOv8 algorithm has demonstrated significant improvements in model size, computation, and parameter count while maintaining high detection accuracy, making it effective for fabric defect detection.
A new lightweight defect detection algorithm, EAL-YOLO, has been introduced to enhance accuracy in complex environments, particularly for small target detection.
The slim-neck structure of EAL-YOLO is designed to improve small target detection while minimizing computational load and model complexity.
This study emphasizes the importance of balancing model accuracy with computational efficiency, particularly for practical applications in real-time monitoring environments.
Experimental results indicate that GSL-YOLOv8 achieved a remarkable reduction in parameters from 26.15 million to 0.61 million and a decrease in computational load from 118.95 GFLOPS to 1.49 GFLOPS, while improving mean Average Precision (mAP) from 96.8% to 98%.
Recent advancements in artificial intelligence have led to the development of intelligent algorithms that significantly enhance fault diagnosis accuracy.
Fixed camera installations combined with computer vision technology are increasingly utilized for real-time hazard detection, addressing the inefficiencies of traditional inspection methods.
Traditional inspection methods, including human visual checks and specialized equipment, are often inefficient and prone to errors, highlighting the advantages of using drones and deep learning technologies.
Future research will focus on enhancing model robustness and implementing real-time diagnostic capabilities through lightweight programs and semi-supervised learning methods.
The YOLO-LSDW model has been optimized for real-time hazard detection in transmission line corridors, achieving a high frame rate of 96.2 FPS, which indicates its suitability for real-time applications.
The enhanced YOLOv8 model integrates an infrared image Slicing-Aided Hyper-Inference (SAHI) technique and Dynamic Snake Convolution (DSConv) to improve detection performance for inter-turn short circuit fault trajectories.
Summary based on 6 sources