Revolutionizing Wind and Solar: Advanced Data Techniques Boost Fault Diagnosis and Efficiency
September 1, 2024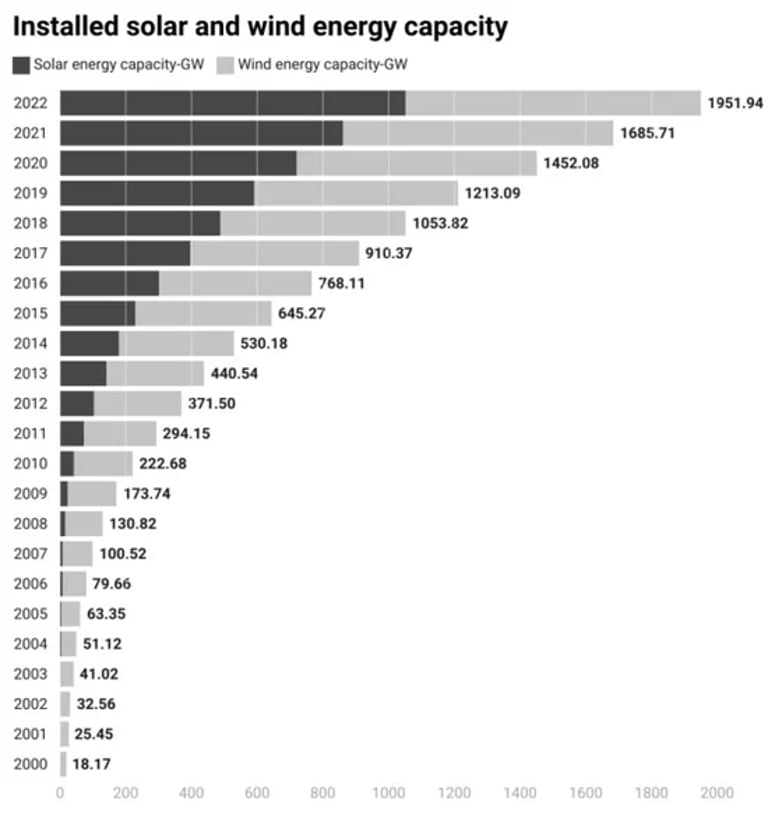
Recent advancements in data stream classification techniques have been applied to wind and photovoltaic power generation, addressing the challenges posed by data drift.
Fault diagnosis is essential in photovoltaic (PV) power plants, particularly due to their vulnerability to complex environmental conditions.
The authors provide guidelines for choosing appropriate OD methods based on the specific detection challenges and characteristics of the data.
The exponentially weighted moving average (EWMA) control chart is effective in detecting abnormal states, such as open and short circuits, and can identify the specific string where a fault occurs.
Streaming data is characterized by concept drift and evolution, complicating traditional data processing methods.
Data drift, which refers to changes in data distribution over time, presents significant challenges for machine learning models and can be categorized into covariate drift, prior probability drift, and concept drift.
Wind turbine generators often face significant downtimes due to manufacturing errors and harsh environmental conditions, which adversely affect their operational efficiency.
The rapid growth of data generated from clean energy sources like wind and solar power necessitates efficient methods to extract valuable insights from these data streams.
Effective management of power station data in photovoltaic systems is crucial for improving operational efficiency and reducing power generation costs.
To enhance fault diagnosis and maintenance strategies in both wind and photovoltaic systems, comprehensive monitoring solutions that analyze component-level data are necessary.
Selecting computationally efficient outlier detection (OD) methods tailored for specific applications is crucial, especially considering the availability of empirical data.
Existing fault diagnosis methods for wind turbines leverage both electrical indicators and vibration signals, emphasizing the role of machine learning for enhanced accuracy.
Summary based on 3 sources