New Study Reveals Data Augmentation's Varied Impact on Machine Learning Model Bias and Performance
August 31, 2024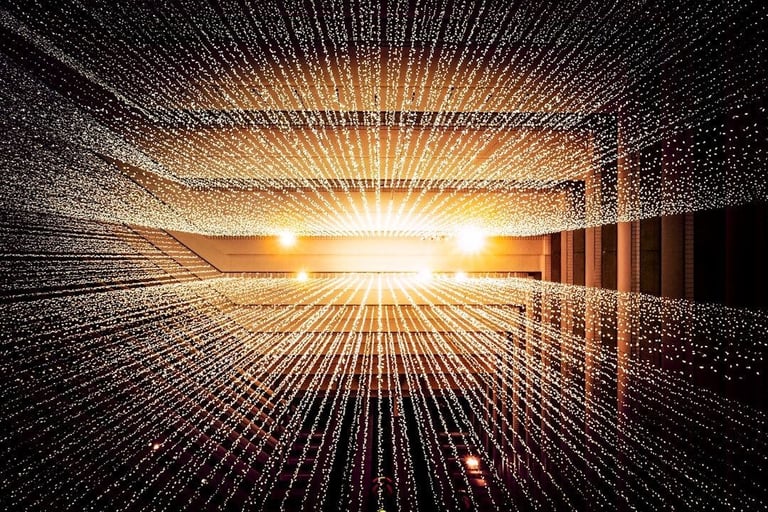
The study, authored by Athanasios Angelakis from Amsterdam University Medical Center and Andrey Rass from Den Haag, Netherlands, investigates the effects of data augmentation (DA) on class-specific bias in machine learning models.
Its overarching goal is to enhance understanding of DA's impact on model performance and bias, contributing to the development of equitable and effective computer vision systems.
The research highlights the importance of understanding potential pitfalls when applying data augmentation to computer vision tasks.
Data augmentation techniques, such as random cropping, stretching, and color jitter, are commonly used to combat overfitting in image-based tasks.
Experiments were conducted using three datasets: Fashion-MNIST, CIFAR-10, and CIFAR-100, with Random Cropping and Random Horizontal Flip augmentations applied.
The ResNet50 architecture was utilized without pre-trained weights to ensure training from scratch, while EfficientNetV2S and SWIN Transformer architectures were also evaluated.
The researchers found that residual models like ResNet50 displayed similar bias effects, while Vision Transformers, particularly SWIN, demonstrated greater robustness.
Results indicated that removing Random Horizontal Flip (RHF) raised the thresholds for optimal class-specific and overall performance, with notable improvements in certain classes.
The study revealed a strong label-erasing effect from excessive DA application, which varied significantly between different classes within the datasets.
The study acknowledges limitations regarding the variety of architectures and datasets tested and encourages future research to explore a broader range of models and data characteristics.
Future research directions include testing additional architectures and datasets, particularly regarding different patch sizes for vision transformers and exploring Capsule Networks.
The findings support previous research by Balestriero, Bottou, and LeCun (2022), indicating that different architectures show consistent performance regarding data augmentation effects.
Summary based on 8 sources