Breakthrough AI Tool Boosts Early Parkinson's Detection with Dual-Channel Technology
August 31, 2024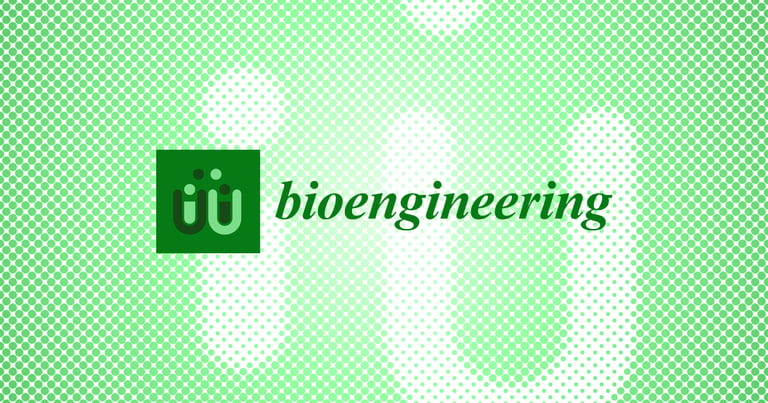
The model also showed higher precision, recall, F1-score, and AUC compared to single-channel metrics, demonstrating its effectiveness.
The research emphasizes the critical importance of early detection of speech impairments in PD, which can manifest significantly before other motor symptoms.
The new CNXV2-DANet model was benchmarked against single-channel settings and three established networks: ConvNeXtV2, ConvNeXt, and Swin Transformer.
Statistical analysis revealed that the dual-channel CNXV2-DANet significantly outperformed all other networks tested, with all p-values below 0.001.
Results indicated that the dual-channel CNXV2-DANet achieved superior performance metrics, boasting an accuracy of 0.839 ± 0.028.
These findings suggest that the dual-channel CNXV2-DANet could enhance TCS-based PD assessment within the medical community.
A novel dual-channel AI-empowered classification tool for Parkinson's disease (PD) has been developed, utilizing transcranial sonography (TCS).
While TCS assesses hyper-echogenicity in the substantia nigra of the midbrain, its subjective nature has previously limited its clinical application.
The researchers employed a dataset of 1,176 TCS images from 588 subjects sourced from Beijing Tiantan Hospital.
This dataset was meticulously divided into training, validation, and testing sets, following a 70%/15%/15% ratio.
Overall, the study supports the integration of advanced machine learning techniques with voice analysis to improve early detection and monitoring of Parkinson's disease.
The article is structured into sections covering introduction, related work, methodology, results, discussion, and conclusion, providing a comprehensive overview of the study.
Summary based on 2 sources