NVIDIA Unveils Game-Changing CUDA Libraries, Boosting Speed and Energy Efficiency for AI and Quantum Computing
August 27, 2024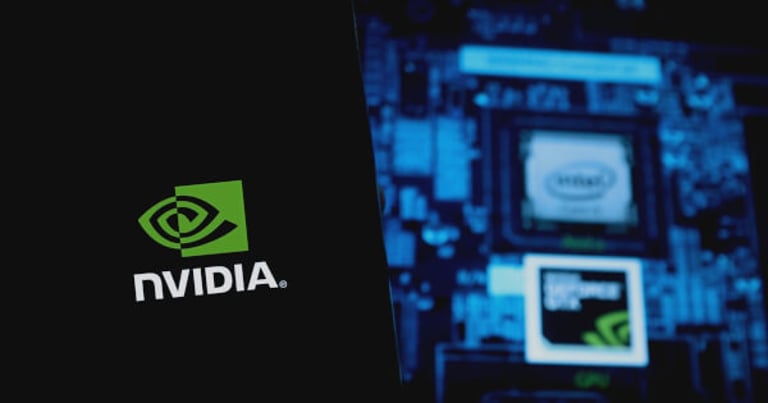
This platform enables large-scale simulations or deployment on physical quantum processing units, enhancing the capabilities of quantum machine learning.
In a notable case, a video conferencing application achieved a remarkable 66x speedup in live captioning throughput after migrating from CPUs to NVIDIA GPUs.
Similarly, an e-commerce platform reported a 33x speedup and nearly 12x improvement in energy efficiency after switching its recommendation system to NVIDIA's cloud computing system.
The recent updates to CUDA libraries include enhancements for large language model applications, data processing, and physical AI simulations, with tools like the cuVS library significantly reducing indexing times.
The integration of these GPU-accelerated libraries into cloud computing platforms not only supports sustainable computing but also offers cost savings for businesses.
As companies increasingly adopt NVIDIA's accelerated computing solutions, they are transitioning from CPU-only applications, leading to substantial gains in both speed and energy efficiency.
NVIDIA has introduced significant advancements in quantum computing through its CUDA-Q platform, previously known as CUDA Quantum, which allows for the development and simulation of novel quantum machine learning (QML) implementations.
CUDA-Q has successfully reduced the resource requirements for quantum clustering algorithms, making them more practical for near-term quantum computing applications.
NVIDIA has launched new CUDA libraries aimed at enhancing accelerated computing, promising significant improvements in speed and energy efficiency across various applications.
NVIDIA estimates that if all AI and data analytics workloads were transitioned to CUDA GPU-accelerated systems, data centers could save approximately 40 terawatt-hours of energy annually, equivalent to the energy consumption of 5 million U.S. homes.
Over the past decade, NVIDIA's AI computing has achieved approximately 100,000x more energy efficiency in processing large language models compared to previous technologies.
NVIDIA NIM offers researchers a streamlined path to deploy machine learning pipelines using optimized containers that integrate multiple libraries and models.
Summary based on 4 sources
Get a daily email with more AI stories
Sources
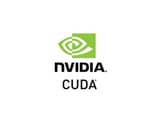
Datanami • Aug 26, 2024
NVIDIA Launches New CUDA Libraries for Accelerated LLM Data Curation and Generation