New TALL Framework Revolutionizes Machine Learning, Boosts Performance for Niche and Mainstream Users Alike
August 21, 2024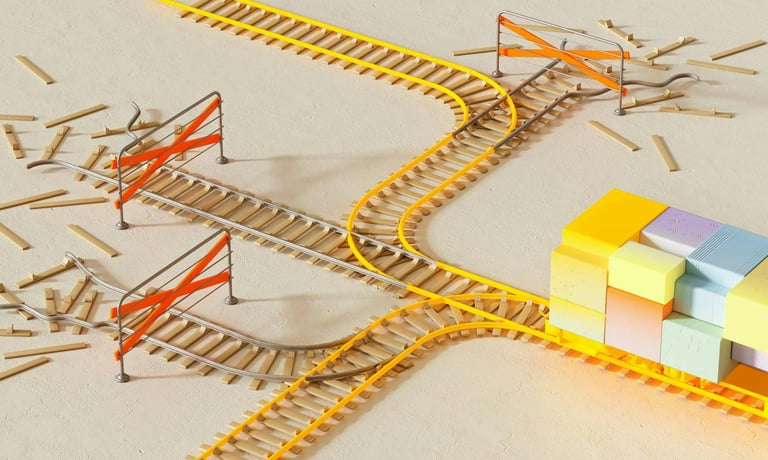
The study introduces the End-to-End Adaptive Local Learning (TALL) framework, designed to debias machine learning models by creating customized local models for different users.
This framework addresses mainstream bias in recommender systems, which negatively affects niche users with minority interests, by focusing on performance differences across various user types.
Mainstream bias arises from two primary issues: discrepancy modeling and unsynchronized learning, complicating recommendations for niche users.
To tackle these challenges, the TALL framework incorporates an adaptive weight module that synchronizes learning paces among users, dynamically adjusting learning rates to improve performance.
The authors aim to enhance utility for niche users while maintaining performance for mainstream users, aligning with the Rawlsian Max-Min fairness principle.
Extensive experiments demonstrate that TALL significantly improves performance for both niche and mainstream users compared to state-of-the-art alternatives.
Experimental results indicate that TALL outperforms existing models, achieving a 6.1% increase in utility for niche users while maintaining model complexity.
A comparative analysis shows that TALL provides the highest NDCG@20 improvement across all datasets and user subgroups, showcasing its efficacy as an end-to-end local learning method.
Key components of TALL include an adaptive loss-driven gate module and an adaptive weight module, which are crucial for its success.
The framework also introduces a gap mechanism to stabilize losses during the initial training phase, enhancing model performance.
The proposed synchronization method balances the performance trade-off between mainstream users, who learn faster, and niche users, who may require a slower learning pace.
The research was conducted by a collaborative team from Texas A&M University and George Mason University, highlighting a significant effort to advance local learning methodologies.
Summary based on 11 sources