ProcTag Revolutionizes Document VQA with Enhanced Training Efficiency and Superior Performance
July 24, 2024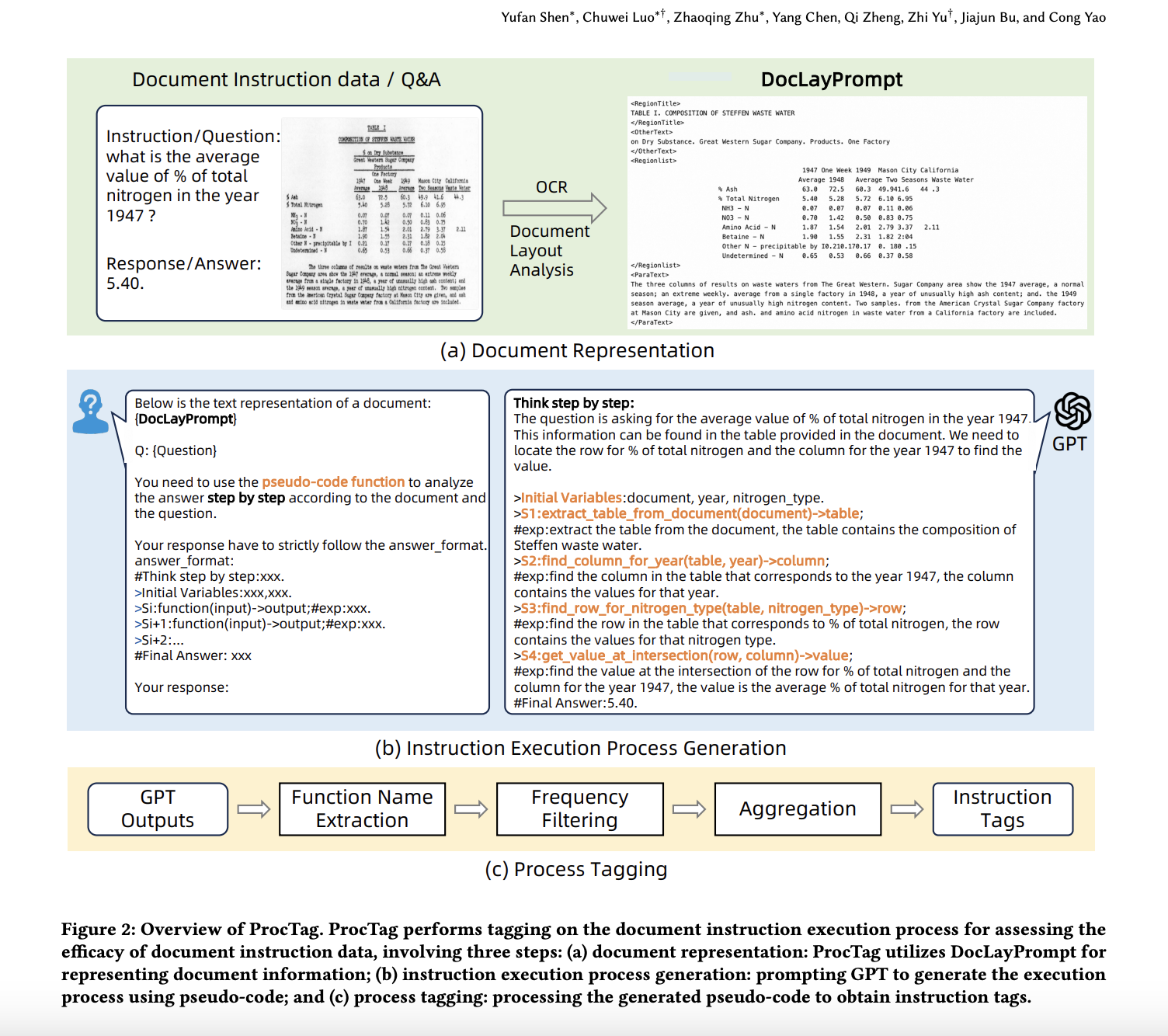
Document Visual Question Answering (DocVQA) focuses on answering queries about document contents like scanned photographs, PDFs, and digital documents.
Visual Question Answering (VQA) combines computer vision and natural language processing to answer questions about images.
ProcTag, proposed by researchers from Alibaba Group and Zhejiang University, shifts focus to the execution process of document instructions, improving data evaluation by tagging the execution process for diversity and complexity.
ProcTag and DocLayPrompt innovations offer a more accurate and efficient approach to training LLMs and MLLMs for document VQA, addressing the limitations of text-based evaluation methods and advancing AI in document understanding.
Existing methods in document instruction data evaluation for training LLMs and MLLMs face challenges focusing only on text and not on the execution process, impacting model performance in document VQA.
ProcTag integrates DocLayPrompt, a layout-aware strategy, to enhance document representation and improve training efficiency and performance of LLMs and MLLMs in document VQA tasks.
The method involves structuring the instruction execution process by using DocLayPrompt to represent documents, generating step-by-step pseudo-code with GPT-3.5, and tagging for diversity and complexity to filter high-efficacy data.
Experimental results show that ProcTag outperforms existing methods like InsTag and random sampling, achieving superior efficacy with minimal data, such as utilizing only 30.5% of the DocVQA dataset for full efficacy.
Docmatix is a monumental DocVQA dataset with 2.4 million pictures and 9.5 million Q/A pairs from 1.3 million PDF documents, significantly larger than previous datasets.
Docmatix was created using Phi-3-small model, ensuring dataset quality by removing hallucinated Q/A pairs and enabling easy access to processed images.
Researchers encourage the open-source community to utilize Docmatix to train new DocVQA models and reduce the disparity between proprietary and open-sourced VLMs.
Training on a subset of Docmatix resulted in a 20% relative improvement in model performance, reducing the performance gap between proprietary and open-source VLMs.
Summary based on 3 sources
Get a daily email with more AI stories
Sources
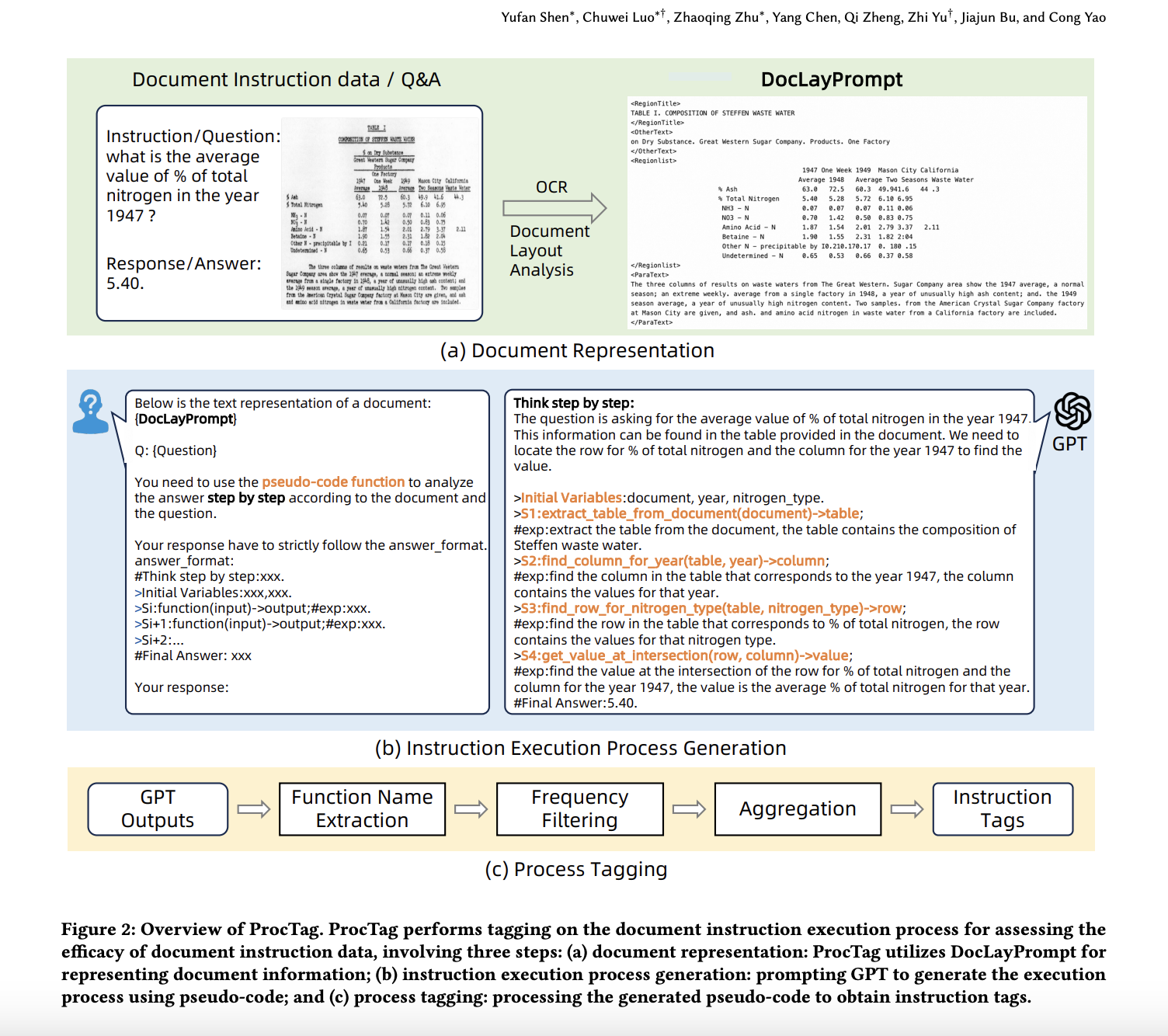
MarkTechPost • Jul 23, 2024
ProcTag: A Data-Oriented AI Method that Assesses the Efficacy of Document Instruction Data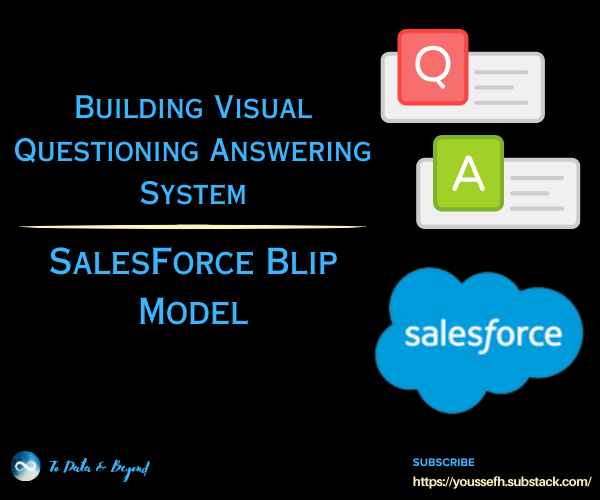
Towards AI • Jul 23, 2024
Building Visual Questioning Answering System Using Hugging Face Open-Source Models