RSG-YOLO Revolutionizes UAV Detection with Enhanced Accuracy and Reduced Computational Load
December 25, 2024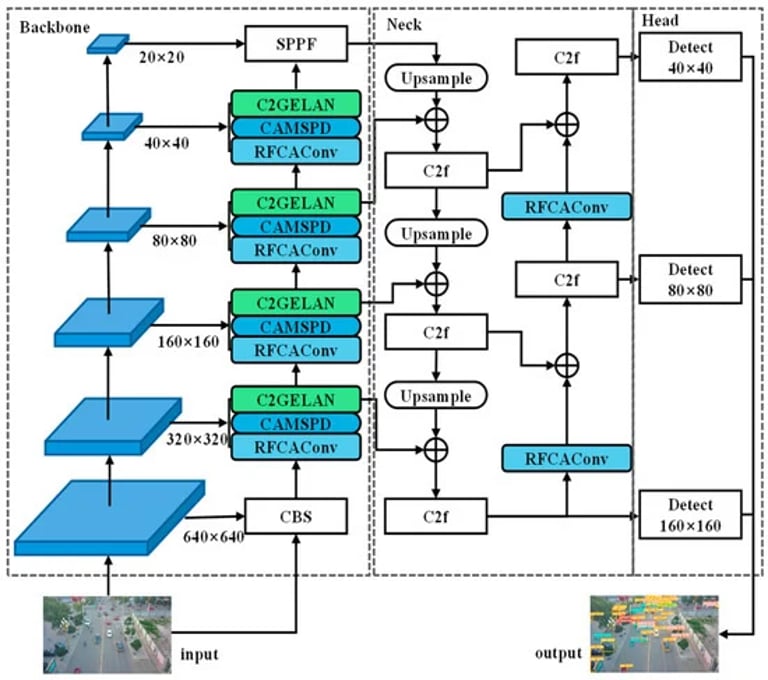
The rapid integration of unmanned aerial vehicles (UAVs) across various sectors, including agriculture, security, and logistics, has prompted research into efficient detection methods due to associated risks to privacy, security, and air traffic safety.
As UAV technology advances, its applications in public safety, wildlife protection, and military operations have increased, yet challenges such as image degradation and background noise complicate detection tasks.
Traditional target detection algorithms often struggle with small target detection due to their reliance on human-designed features, while deep learning algorithms, particularly convolutional neural networks (CNNs), have demonstrated superior efficacy.
Current YOLO-based models are widely used for small target detection on UAVs, but there remains a critical need to optimize the balance between detection speed and accuracy in practical scenarios.
In response to these challenges, a new lightweight model called RSG-YOLO has been proposed, which integrates attention mechanisms and fine-grained downsampling to enhance the detection capabilities of small targets from UAV perspectives while minimizing resource usage.
Key innovations in RSG-YOLO include the Receptive-Field Coordinated Attention (RFCAConv) module, which focuses on critical areas while ignoring background noise, and an improved downsampling structure (CAMSPD) that retains detailed information about small targets.
The model also incorporates a Haar wavelet downsampling (HWD) module for efficient feature extraction with fewer parameters, further enhancing its performance.
Additionally, a lighter Cross-Channel Feature Fusion Module (CCFM) replaces the original neck portion of the model, improving the detection of small UAV targets.
Although there is a 5% decrease in frames per second (FPS), RSG-YOLO shows significant improvements in overall detection performance compared to the baseline YOLOv11 model.
Experimental results using the DUT ANTI-UAV dataset indicate improvements in precision, recall, and mean average precision (mAP) metrics by 4%, 4.5%, and 4.1%, respectively, while reducing parameters by 38.4%.
These results demonstrate that RSG-YOLO achieves a significant reduction in parameters and computational load while improving detection accuracy, showcasing its effectiveness for UAV applications.
The study underscores the necessity of developing efficient UAV detection methods to keep pace with the rapid advancements in UAV technology.
Summary based on 2 sources