Revolutionizing Agriculture: CNNs and Deep Learning Enhance Crop Resilience and Wind Turbine Damage Detection
October 7, 2024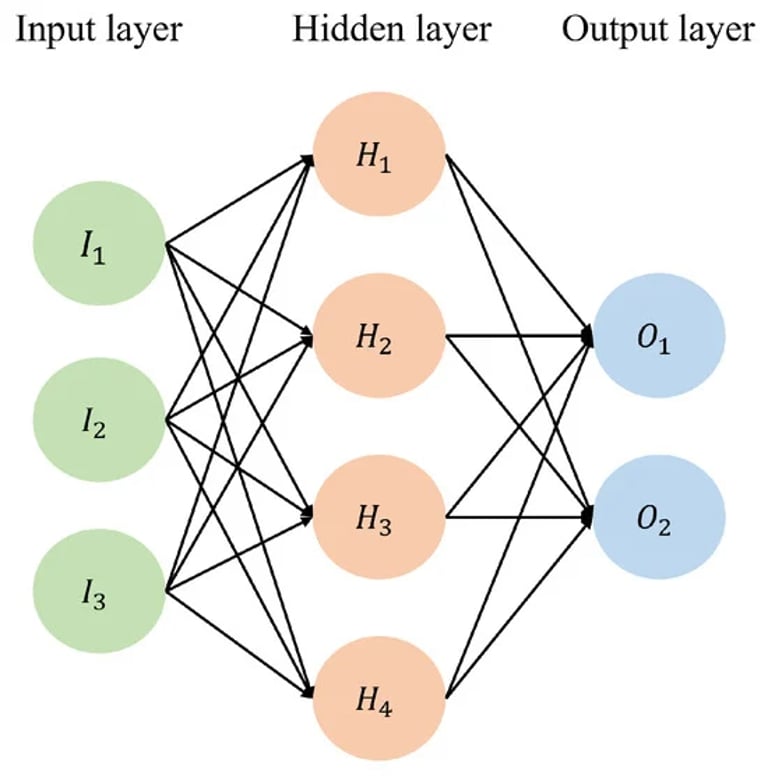
Traditionally labor-intensive and costly, plant phenotyping is being revolutionized by remote sensing technologies, including UAVs and satellites, which enhance data collection efficiency.
The proposed method was validated using datasets from Case Western Reserve University and a wind turbine test bench, demonstrating its applicability in real-world scenarios.
The research focuses on enhancing damage detection in wind turbine blades (WTBs) by addressing current limitations in accuracy and robustness.
Convolutional Neural Networks (CNNs) are becoming essential for automated disease detection in crops, leveraging their capability to learn from extensive image datasets.
Employing a combination of deep learning techniques and a diffusion model framework, the methodology allows for gradual improvements in change detection outcomes.
The ultimate aim is to develop crops that are more resilient to extreme weather, require fewer pesticides, and can significantly boost food production.
Accurate biomass estimation is crucial for sustainable agriculture, especially given the rising food demand and environmental pressures.
The study evaluated multiple versions of the YOLO model, identifying YOLOv9c as the most effective in terms of precision, recall, and mean Average Precision (mAP).
These findings were published in Nature Plants and are grounded in data collected from five experimental fields.
Overall, the research showcases significant advancements in model performance through automated processes, paving the way for more efficient precision agriculture.
This approach effectively manages feature correlations, ensuring that relevant parameters related to yaw system issues are retained.
This innovative approach is particularly significant for the Peruvian Andes, where previous research on forage production has been limited.
Summary based on 77 sources
Get a daily email with more AI stories
Sources
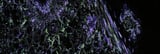
Phys.org • Sep 30, 2024
New method achieves first-ever imaging of pathogens on lettuce leaves in real-time