Revolutionary Multi-Cancer Detection Method Achieves 93% Accuracy with SERS and Deep Learning
February 22, 2025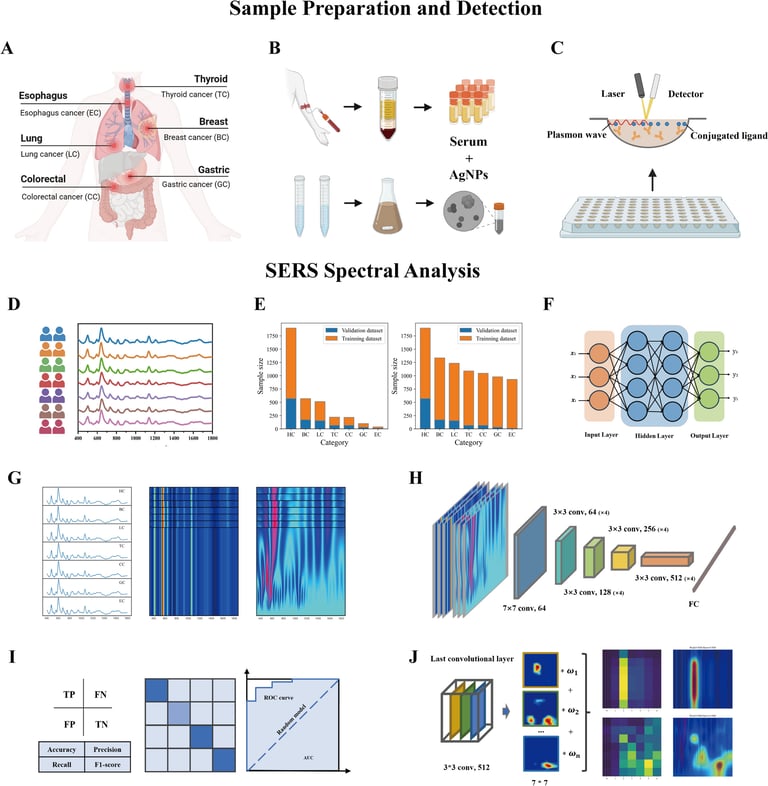
This SERS technology significantly amplifies the Raman scattering signal, which enhances sensitivity and accuracy in detecting various cancers through serum sample analysis.
A recent study has introduced an innovative multi-cancer early detection method that utilizes serum surface-enhanced Raman spectroscopy (SERS) combined with advanced deep learning techniques.
The study involved a substantial cohort of 3,551 participants, including 1,655 individuals with early-stage cancer and 1,896 healthy controls, covering a range of cancers such as breast, lung, thyroid, colorectal, gastric, and esophageal.
The study achieved an impressive accuracy rate of 93.15% and an area under the curve (AUC) value of 0.996 in distinguishing healthy individuals from those with various cancer types.
Furthermore, the study identified key spectral peaks linked to different cancers, revealing distinct biochemical signatures in serum that underscore the potential of SERS for multi-cancer screening.
The results indicate that the SERS-based approach could serve as a cost-effective and efficient early detection strategy for multiple cancer types, potentially transforming clinical screening practices.
To optimize the input for convolutional neural networks (CNNs), the researchers transformed spectral data into two-dimensional images, which enhanced the interpretability of the findings.
To tackle the challenge of an imbalanced dataset, the researchers employed the BorderlineSMOTE method, which proved to be the most effective resampling strategy, significantly boosting classification performance.
Summary based on 1 source