Breakthrough AI Model GET Revolutionizes Gene Activity Prediction Across Human Cell Types
January 8, 2025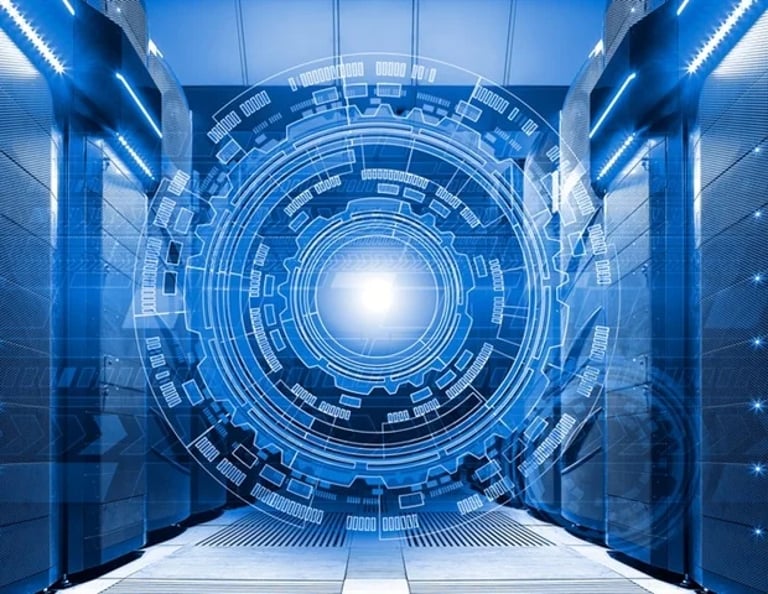
Raul Rabadan, a professor at Columbia and director of the Program for Mathematical Genomics, emphasizes that GET's predictive capability can transform biology from a descriptive science to one that can anticipate cellular behavior and responses to mutations.
This innovative research, published in the journal Nature, signifies a shift towards using AI in biology, heralding a new era of predictive capabilities in the field.
While the 2024 Nobel Prize in Chemistry recognized advancements in AI for predicting protein structures, predicting gene activity within cells has presented greater challenges, which GET aims to overcome.
Rabadan's team is investigating various cancers to illuminate the genetic changes that drive disease mechanisms, representing a shift towards a more predictive science in biology.
The study emphasizes how predictive computational models can enhance traditional experimental approaches and uncover biological processes.
Overall, this research opens new avenues for understanding various diseases and could lead to the identification of new treatment targets.
Researchers at Columbia University Vagelos College of Physicians and Surgeons have developed a groundbreaking artificial intelligence model called General Expression Transformer (GET) that predicts gene activity across various human cell types.
Unlike previous models that focused on cancer cell lines, GET was trained on gene expression data from over 1.3 million normal human cells, allowing it to predict gene expression in previously unseen cell types with high accuracy.
Traditionally, biological research has focused on describing cell functions and responses, but GET enables predictions about cell activities and reactions to changes, such as cancer-causing mutations.
The model also explores the genome's 'dark matter,' referring to non-coding regions that may harbor mutations affecting cancer progression.
In a case study on pediatric leukemia, GET successfully predicted how mutations affect transcription factors, with findings confirmed through laboratory experiments.
GET may streamline research by prioritizing experiments that are most likely to yield useful results, addressing the overwhelming number of genetic combinations in diseases like cancer.
Summary based on 4 sources
Get a daily email with more AI stories
Sources
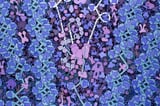
The Washington Post • Jan 8, 2025
Scientists trained AI to predict gene activity, a potentially powerful tool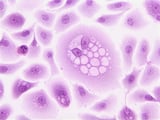
Phys.org • Jan 8, 2025
Computational biologists develop AI that predicts inner workings of cells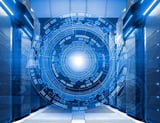
News-Medical • Jan 9, 2025
New AI method predicts gene activity in human cells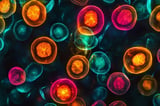
Neuroscience News • Jan 8, 2025
AI Reveals Gene Activity in Human Cells - Neuroscience News