Revolutionary AI-Driven Breakthrough Boosts Perovskite Solar Cell Efficiency to Near-Record Levels
December 20, 2024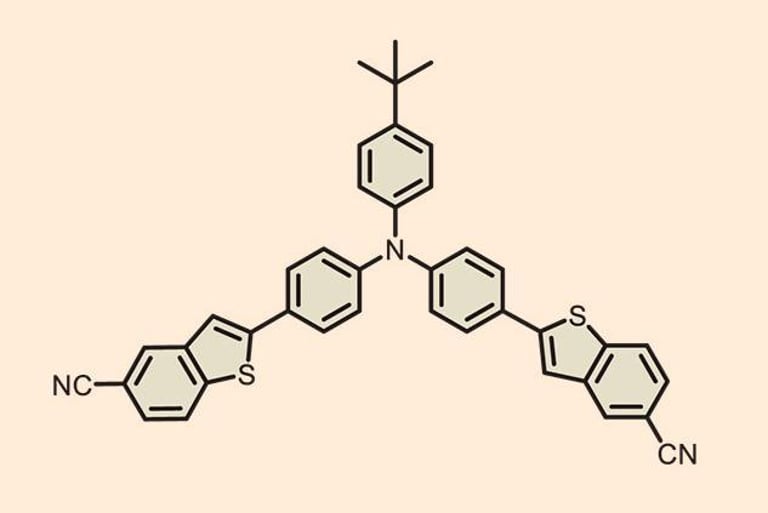
An international team of researchers from the University of Erlangen–Nuremberg, Karlsruhe Institute of Technology, and Ulsan National Institute of Science and Technology has developed a hole-transporting layer for perovskite solar cells using a machine learning algorithm.
This innovative study employed a machine-learning algorithm to sift through a dataset of over one million candidates, ultimately narrowing it down to 101 selected molecules.
From this analysis, the algorithm suggested 24 promising candidates for further testing, highlighting the potential for rapid exploration of chemical space critical for solar technology.
The focus on the hole-transporting layer is crucial, as it plays a significant role in transporting holes to the positive electrode, directly impacting the overall power conversion efficiency of solar cells.
Despite their importance, effective hole-transporting materials have been limited in commercial applications, often discovered through experimental methods rather than a theoretical understanding.
The synthesized solar cells achieved power conversion efficiencies of up to 26.2%, coming close to the current record of 26.7%, suggesting that the team's approach could lead to further advancements in solar cell technology.
The researchers plan to extend their machine-learning approach to optimize the electron-transport layer and ultimately enhance the entire solar cell structure.
Experts Ted Sargent and Cheng Liu emphasized the significance of this work as a major advance in applying machine learning to perovskite photovoltaics, showcasing a practical method for accelerating materials discovery.
Pascal Friederich expressed optimism that the successful identification of multiple materials could enhance the theoretical understanding of perovskite solar cells.
The findings were published on December 19, 2024, in the journal Science, authored by Jianchang Wu and colleagues, marking a significant contribution to the field.
This research not only demonstrates near-record performance but also opens the door for future discoveries that could surpass existing efficiency records.
Overall, this study represents a pivotal step forward in the quest for more efficient solar energy solutions, leveraging machine learning to revolutionize material discovery.
Summary based on 2 sources
Get a daily email with more AI stories
Sources
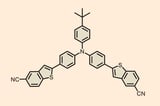
Chemistry World • Dec 18, 2024
AI aids discovery of solar cell materials with near-record efficiency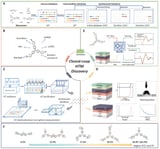
Tech Xplore • Dec 19, 2024
Machine learning helps researchers develop perovskite solar cells with near-record efficiency