Breakthrough Photonic AI Chips Outperform GPUs, Slashing Energy Use and Boosting Efficiency
April 14, 2025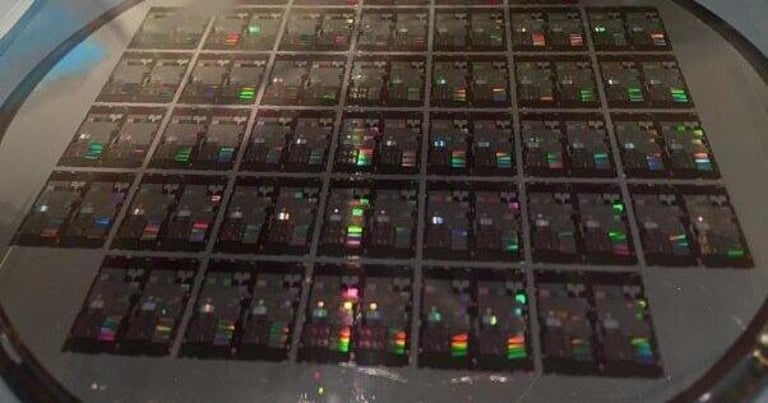
The photonic platform achieves wafer-scale integration of essential components for an optical neural network on a single chip, significantly improving data center capabilities for AI workloads.
A recent study published in the IEEE Journal of Selected Topics in Quantum Electronics reveals that photonic integrated circuits (PICs) utilizing III-V compound semiconductors can execute AI workloads more efficiently than traditional GPU-based architectures.
The fabrication of this innovative hardware begins with silicon-on-insulator (SOI) wafers and employs advanced techniques such as lithography, doping, and selective growth of silicon and germanium for photodetectors.
By integrating silicon photonics with III-V compound semiconductors, the platform enables the development of efficient on-chip lasers, amplifiers, and optical components.
The researchers have developed a new AI acceleration hardware platform that significantly reduces energy requirements while effectively handling large workloads.
This advancement aims to address the computational and energy challenges in AI, paving the way for more robust and sustainable AI accelerator hardware in the future.
Led by Dr. Bassem Tossoun from Hewlett Packard Labs, the research highlights the superior scalability and energy efficiency of photonic AI accelerators compared to existing electronic distributed neural networks (DNNs).
This fabrication process incorporates a heterogeneous integration approach, utilizing die-to-wafer bonding with III-V materials to enhance performance.
This innovative platform can integrate all necessary components for building optical neural networks (ONNs) on a single chip, achieving energy efficiency that is 2.9 times greater than other photonic platforms and 1.4 times greater than current digital electronics.
The increasing processing demands of AI for training models have created challenges related to energy costs and processing capabilities in infrastructures that rely heavily on GPUs.
Photonic AI accelerators leverage optical neural networks (ONNs) that process data at the speed of light, resulting in minimal energy loss compared to traditional electronic DNNs.
Dr. Tossoun emphasizes that while silicon photonics are easy to manufacture, scaling them for complex circuits is challenging; however, their new platform overcomes this limitation, providing a foundation for more efficient photonic accelerators.
Summary based on 4 sources
Get a daily email with more AI stories
Sources
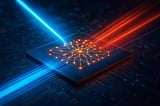
SciTechDaily • Apr 14, 2025
AI at the Speed of Light: How Silicon Photonics Are Reinventing Hardware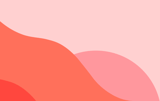
HPCwire • Apr 14, 2025
IEEE Study Leverages Silicon Photonics for Scalable and Sustainable AI Hardware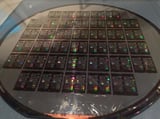
Dataconomy • Apr 13, 2025
New IEEE study explores AI acceleration with photonics - Dataconomy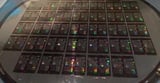
Curated - BLOX Digital Content Exchange • Apr 11, 2025
IEEE Study Leverages Silicon Photonics for Scalable and Sustainable AI Hardware