Google DeepMind Study Reveals AI Models Surpass Humans in Factual Accuracy with New Evaluation Framework
April 9, 2025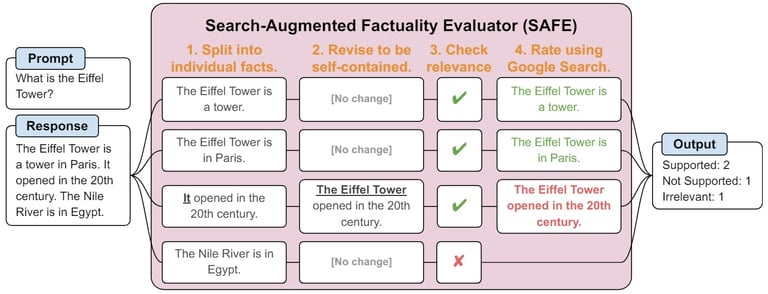
LongFact consists of 2,280 fact-seeking prompts covering 38 topics, created with the help of GPT-4, to benchmark the long-form factuality of model responses.
The study identifies future directions for improving language model factuality through better pretraining or finetuning techniques, while also addressing the issue of hallucination in model outputs.
Key contributors to the research include experts from Google DeepMind, highlighting a collaborative effort in advancing language model capabilities.
Notably, newer models like Claude-3-Opus and Claude-3-Sonnet perform comparably to or better than GPT-4, indicating advancements in model architecture.
A new study by Google DeepMind evaluates the factual accuracy of 13 major AI language models, including GPT-4, Gemini-Ultra, and PaLM-2-L-IT-RLHF, using a framework called LongFact and the Search-Augmented Factuality Evaluator (SAFE).
The research introduces an innovative metric called F1@K, which combines precision and recall to enhance the evaluation of long-form responses by requiring a minimum number of supported facts for full scores.
SAFE is an automated evaluative method that breaks down long responses into individual facts and verifies them using Google Search, showing a 72% agreement with human annotators and outperforming them in 76% of disagreement cases.
The total cost for SAFE to evaluate 496 pairs was $96.31, making it over 20 times cheaper than crowdsourced human annotations, which cost $4 per model response.
Claude-3-Sonnet's performance is particularly interesting as it matches that of the larger Claude-3-Opus model despite being smaller, suggesting potential efficiency in design.
The study notes that while factual recall has improved significantly with model scaling, improvements in factual precision have not kept pace.
While human annotators are common in language-model research, their involvement often leads to bottlenecks due to high costs and variability in evaluation quality.
LLMs, particularly through the SAFE framework, have demonstrated superhuman performance in reasoning and higher factuality than humans on summarization tasks.
Summary based on 4 sources
Get a daily email with more Tech stories
Sources
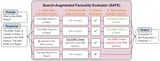
HackerNoon • Apr 8, 2025
The AI Truth Test: New Study Tests the Accuracy of 13 Major AI Models