Revolutionary ICPL Method Surpasses Traditional Reinforcement Learning with Human Feedback in Efficiency and Performance
December 4, 2024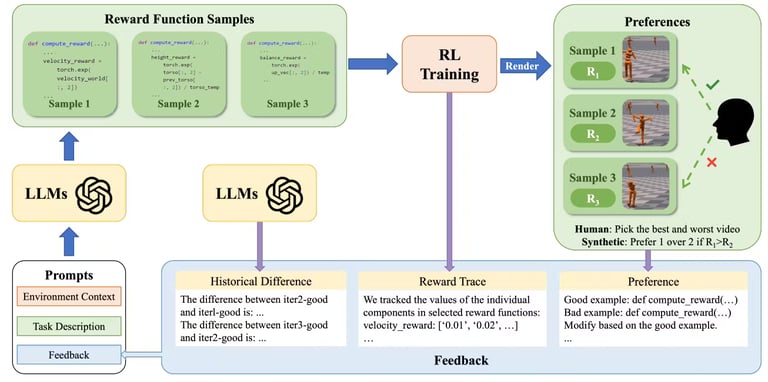
The research included two experimental sets: one using proxy human preferences derived from human-designed rewards, and the other involving real human participants to evaluate the method's practical effectiveness.
In the Humanoid task, iterations adjusted reward components to optimize the speed of a humanoid robot, demonstrating the iterative nature of ICPL in enhancing performance.
Validation of ICPL's design was achieved through ablation studies, confirming the importance of components like reward traces in improving performance across various tasks.
The article introduces In-Context Preference Learning (ICPL), a novel approach that utilizes large language models (LLMs) to autonomously generate reward functions for preference learning tasks.
Experimental results demonstrate that ICPL significantly outperforms traditional reinforcement learning from human feedback (RLHF) in efficiency and competes effectively with methods relying on ground-truth rewards.
ICPL operates by synthesizing reward functions based on task descriptions and environmental context, iteratively refining these functions through human evaluations of agent behaviors.
Human-in-the-loop experiments revealed that while consistency in real human feedback was challenging, it positively influenced the refinement of reward functions, yielding better outcomes than the OpenLoop method.
ICPL showcased a remarkable reduction in the number of required preference queries, achieving similar or superior performance compared to traditional preference-based RL algorithms.
The method enhances efficiency by directly searching for optimal reward functions using LLMs, eliminating the need for extensive human feedback typically required in traditional RLHF.
The collaborative research effort involved authors from Tsinghua University and New York University, emphasizing the interdisciplinary approach to advancing preference-based reinforcement learning methodologies.
Recent advancements in the field have explored the use of LLMs and vision-language models to generate reward signals, indicating a growing trend towards integrating AI in reward design.
The study highlights the effectiveness of human-in-the-loop reinforcement learning as a method for aligning agent behaviors with human preferences, supported by recent research findings.
Summary based on 6 sources