Federated Learning Revolutionizes IoT for Safer, More Efficient Connected Vehicles
September 14, 2024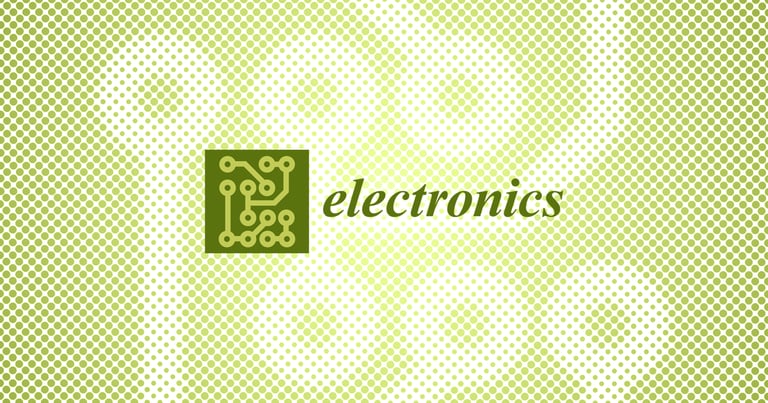
However, privacy concerns and communication costs associated with cloud-based data processing present challenges that hinder the full realization of IoT benefits.
This approach allows multiple devices to coordinate with a central server for data training while ensuring data privacy.
Federated learning (FL) has emerged as a promising solution to these issues, enabling edge devices to collaboratively train models without sharing sensitive data.
The Internet of Things (IoT) is revolutionizing various sectors by facilitating data sharing and decision-making among connected devices, which generates vast amounts of data.
The proposed framework addresses challenges such as multimodal data integration, Byzantine attacks, and communication constraints due to vehicle mobility.
Moreover, the proposed strategy enhances content caching based on data popularity, leading to improved network performance.
Recent research has focused on developing a Byzantine-robust multimodal federated learning framework to enhance Intelligent Connected Vehicles (ICVs).
ICVs utilize diverse data sources, including sensors and Vehicle-to-Everything (V2X) communications, to improve road safety and traffic efficiency.
In particular, the introduction of the Uncertainty-Aware Federated Reinforcement Learning (UA-FedRL) method optimizes performance by dynamically selecting local epochs for IoT devices.
Key contributions of this research include a novel aggregation technique based on gradient compression, advanced multimodal data fusion, and improved communication efficiency.
Comparative analysis indicates that the new caching strategy significantly outperforms existing benchmarks in cache hit ratio, content retrieval delay, and energy consumption.
This method pairs with a Predictive Weighted Average Aggregation (PWA) approach to tackle weight aggregation challenges across heterogeneous devices.
Summary based on 3 sources