Revolutionary Algorithm Combines NIR and Deep Learning to Perfect Wood Defect Detection and Enhance Manufacturing Quality
September 1, 2024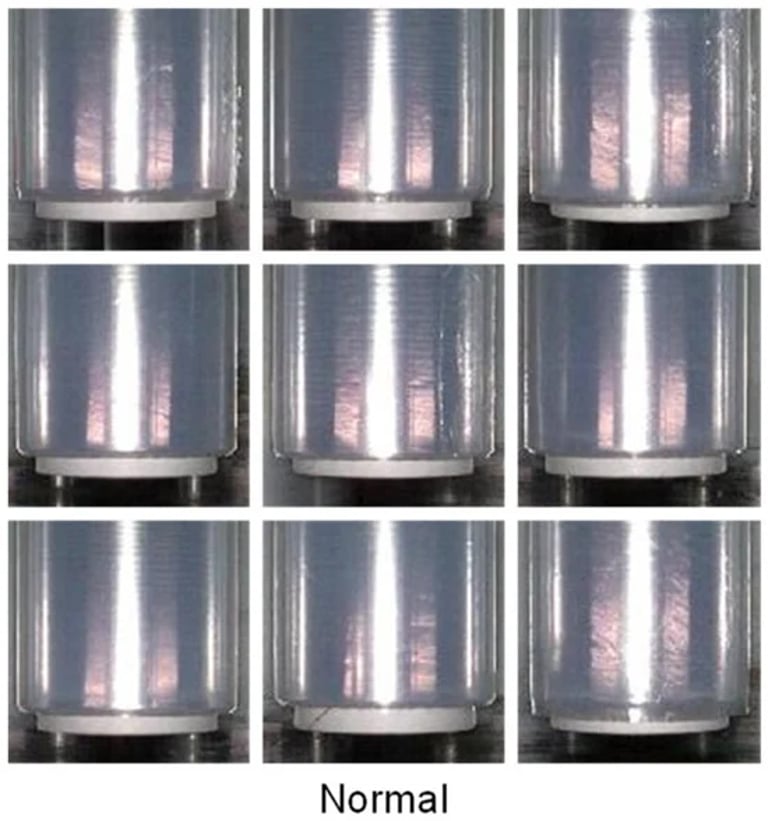
Accurate detection of wood defects is essential for enhancing wood utilization efficiency and reducing carbon emissions by maximizing mechanical properties.
A recent study has introduced the WD-1D-VGG19-FEA algorithm, which combines near-infrared (NIR) spectroscopy with a one-dimensional VGG19 deep learning model to detect wood defects and predict mechanical properties.
Finite element analysis (FEA) was utilized to create a nonlinear three-dimensional model of defective wood, predicting the elastic modulus with a low error margin.
Recent advancements in deep learning, especially with algorithms like YOLO, have significantly improved the rapid and accurate quality detection capabilities in manufacturing.
Traditional manual inspection methods often introduce subjectivity and inconsistency, highlighting the need for automated solutions to improve quality control.
Comparative results indicated that the VGG19 model outperformed other classification algorithms in accuracy, making it a preferred choice for complex data classification.
NIR spectroscopy is recognized as a non-destructive and efficient method for understanding the internal structure and defects of wood, which is vital for improving its mechanical properties.
The study involved analyzing 1080 sets of NIR data from various wood regions, achieving high accuracy in identifying knot areas, fiber deviations, and transition areas.
The study also presents a novel approach that combines Convolutional Neural Networks (CNNs) with One-Class Support Vector Machines (OCSVMs) to enhance defect detection in wrap film products.
The manufacturing industry of wrap film products faces challenges in quality control, necessitating advanced defect detection methods to address the delicate nature of these products.
To tackle data imbalance in training sets, Generative Adversarial Networks (GANs) were employed to synthesize defective samples.
The study emphasizes the careful adjustment of parameters in OCSVMs to balance false positive and false negative rates during anomaly detection.
Summary based on 3 sources