Amazon Bedrock Advances AI with Cost-Effective Context Caching and Customization Tools
August 6, 2024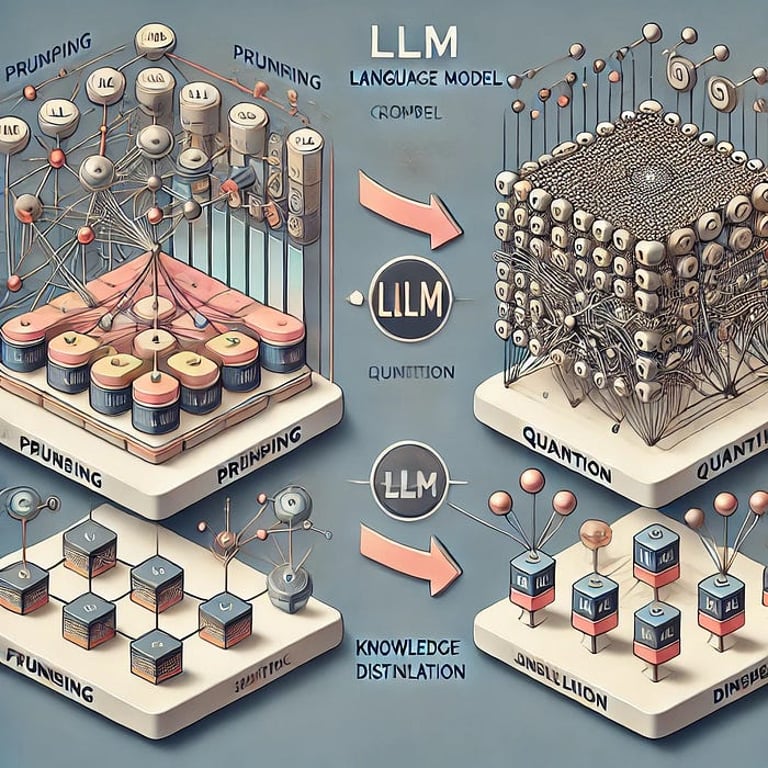
This week, significant advancements in large language model (LLM) inference were observed, particularly with context caching, which has notably reduced costs for reused input tokens.
These advancements in context caching and reduced inference costs are expected to enhance the economic viability of LLM systems, particularly in multi-shot learning capabilities.
Amazon Bedrock is providing a customizable foundation for generative AI applications, enabling businesses to tailor models to meet their specific needs.
Customization techniques available in Amazon Bedrock include prompt engineering, retrieval augmented generation (RAG), and model fine-tuning, which can be applied to both labeled and unlabeled data.
Generative AI models are transforming software development workflows by enabling automated application generation, which streamlines the traditional software development life cycle (SDLC) through natural language prompts.
The integration of generative AI into the SDLC can lead to significant productivity gains, making it essential for teams to adopt these technologies.
Retrieval Augmented Generation (RAG) enhances AI system reliability by allowing LLMs to consult authoritative external knowledge bases, thereby improving the relevance and accuracy of responses.
Developing modular components within AI systems allows for flexibility and stability, enabling teams to remain competitive in an evolving technical landscape.
After deploying these systems, users can test the solution by initiating data synchronization and querying their documents using natural language.
Recent announcements at the AWS New York Summit included new capabilities for indexing public web pages and advanced chunking options for data retrieval.
Evaluation of LLMs is crucial, and MLflow provides built-in and custom metrics to assess performance and behavior, allowing users to define metrics that evaluate aspects like professionalism in generated responses.
The quality of an LLM's response improves significantly when users provide detailed context, including system and user messages.
Summary based on 9 sources
Get a daily email with more Tech stories
Sources
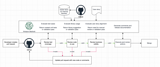
Amazon Web Services • Aug 6, 2024
Use Amazon Bedrock to generate, evaluate, and understand code in your software development pipeline | Amazon Web Services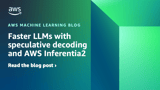
Amazon Web Services • Aug 5, 2024
Faster LLMs with speculative decoding and AWS Inferentia2 | Amazon Web Services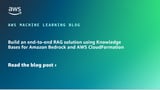
Amazon Web Services • Aug 5, 2024
Build an end-to-end RAG solution using Knowledge Bases for Amazon Bedrock and AWS CloudFormation | Amazon Web Services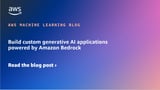
Amazon Web Services • Aug 6, 2024
Build custom generative AI applications powered by Amazon Bedrock | Amazon Web Services