Revolutionizing Cybersecurity: How Machine Learning is Transforming Intrusion Detection and SOC Efficiency
June 11, 2024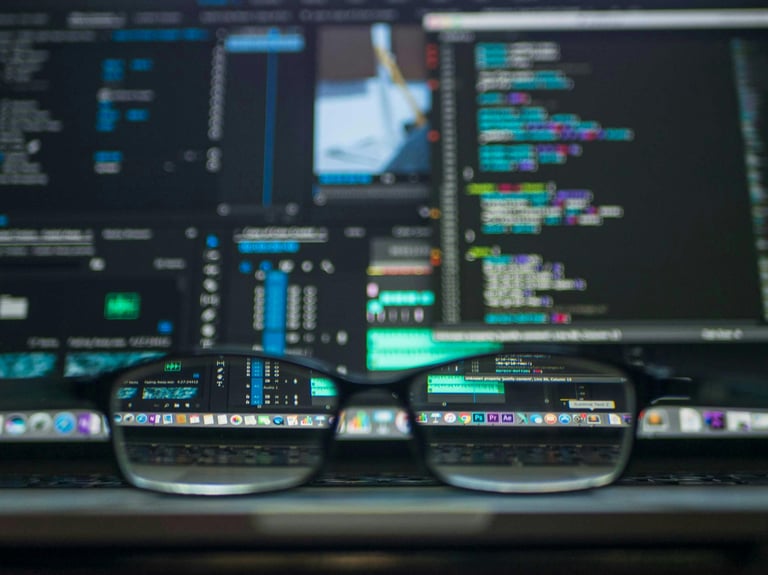
Researchers are using advanced machine learning techniques to enhance Intrusion Detection Systems (IDS) against cyber threats like spam, malware, and network intrusions.
Deep learning methods such as autoencoders and LSTM are improving detection accuracy.
Challenges like imbalanced training data and feature selection highlight the need for a comprehensive defense strategy.
The integration of both AI and non-AI solutions is crucial for effective cybersecurity, as emphasized in a Special Issue on Intrusion and Malware Detection and Prevention.
Security Operations Centers (SOCs) face issues with alert management, including high rates of false positives and alert fatigue.
Machine learning (ML) can help SOCs by adapting to emerging threats, recognizing patterns, and automating tasks to improve efficiency.
ML techniques in SOCs include supervised learning for known threats, unsupervised learning for unknown threats, and reinforcement learning for dynamic environments.
Implementing ML in SOCs requires high-quality data, expertise, workflow integration, and regulatory compliance.
The future of SOCs will be shaped by ML, revolutionizing efficiency, alert tuning, threat detection, and proactive cybersecurity defenses.
Summary based on 3 sources