MIT Study Unveils LLMs' Brain-Like Reasoning, Enhancing Multilingual AI Efficiency
February 20, 2025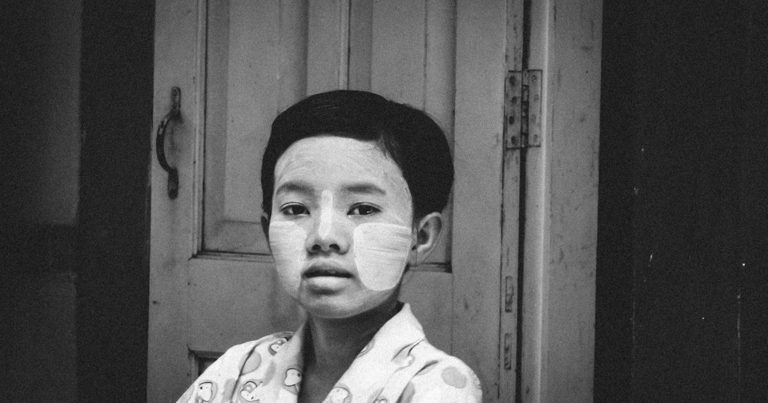
A significant finding shows that English-dominant LLMs convert foreign-language inputs into an English-centric representation for analysis and output generation, indicating a reliance on a dominant linguistic framework.
The findings aim to improve understanding of LLMs' internal mechanisms, which could lead to enhanced training methods for handling diverse data.
Future research could explore how to balance the sharing of knowledge across languages while maintaining language-specific processing abilities, particularly for culturally specific knowledge.
Interventions during model processing demonstrated predictable alterations in outputs, reinforcing the concept of a centralized semantic processing mechanism within LLMs.
The study highlights that LLMs convert specific tokens from their input data into modality-agnostic representations, similar to how the anterior temporal lobe in the human brain functions as a 'semantic hub' to integrate information from various sensory modalities.
Recent research from MIT, partially funded by the MIT-IBM Watson AI Lab, reveals that large language models (LLMs) exhibit reasoning mechanisms akin to the human brain, particularly in their processing of diverse data types.
This research suggests that LLMs generalize data processing by using a dominant language to handle inputs in other languages, mirroring the brain's method of routing information.
By manipulating a model's semantic hub with English text, researchers were able to influence outputs even when processing other languages, indicating a shared knowledge base within the model.
Zhaofeng Wu, a lead author from MIT, emphasizes the need for a better understanding of LLMs to improve and control their performance.
The understanding of LLMs' data integration has important implications for AI development, including enhanced efficiency and improved multilingual processing capabilities.
Insights from this study may help improve multilingual models, preventing accuracy loss in English when learning other languages and enhancing overall performance.
The research demonstrated that LLMs assign similar representations to inputs of different types but with similar meanings, such as text, images, and audio.
Summary based on 3 sources
Get a daily email with more AI stories
Sources
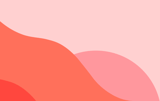
ScienceDaily • Feb 19, 2025
Like human brains, large language models reason about diverse data in a general way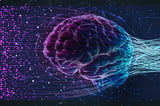
MIT News | Massachusetts Institute of Technology • Feb 19, 2025
Like human brains, large language models reason about diverse data in a general way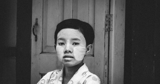
IndiaAI • Feb 21, 2025
The Cognitive Convergence: How AI and Human Brains Process Language