Microsoft's Magma AI Revolutionizes Multimodal Integration, Outperforming Rivals in Robotics and UI Navigation
February 20, 2025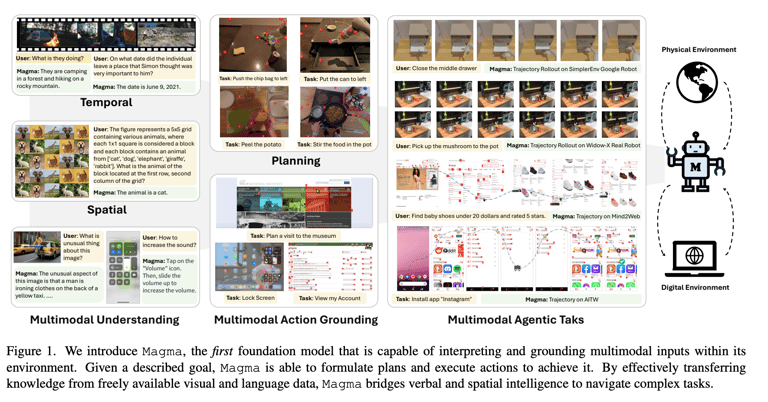
Microsoft researchers have unveiled Magma, a groundbreaking multimodal AI model designed to integrate vision, language, and action, aiming to enhance applications in robotics, UI navigation, and intelligent decision-making.
The model excelled in multimodal understanding, achieving 80.0% accuracy in VQA v2 and showcasing strong spatial reasoning with scores of 74.8% on the BLINK dataset.
Magma seeks to unify existing AI capabilities that typically focus on either vision-language understanding or robotic manipulation into a single adaptable model.
Magma addresses the limitations of current Vision-Language-Action (VLA) systems, which often struggle to generalize across different environments and tasks.
The model utilizes a ConvNeXt-XXL vision backbone for processing images and an LLaMA-3-8B language model for handling text inputs, effectively merging vision-language understanding with action execution.
Magma employs innovative techniques such as Set-of-Mark (SoM) for labeling actionable visual objects and Trace-of-Mark (ToM) for tracking object movements over time, enhancing its planning capabilities.
In robotic manipulation tasks, Magma achieved a success rate of 52.3% in Google Robot tasks and 35.4% in Bridge simulations, significantly outperforming the previous OpenVLA model.
In zero-shot UI navigation tasks, Magma recorded a selection accuracy of 57.2%, surpassing other models like GPT-4V-OmniParser and SeeClick.
Key takeaways from the research highlight Magma's ability to generalize across tasks without requiring additional fine-tuning, indicating its potential to enhance decision-making in various AI applications.
Trained on a diverse dataset comprising 39 million samples, including UI navigation tasks, robotic action trajectories, images, and videos, Magma ensures robust multimodal learning.
In video question-answering tasks, Magma demonstrated impressive performance with an accuracy of 88.6% on IntentQA, highlighting its capability to process temporal information effectively.
Summary based on 1 source