AI in Healthcare: Balancing Efficiency and Ethics in Resource Allocation
December 21, 2024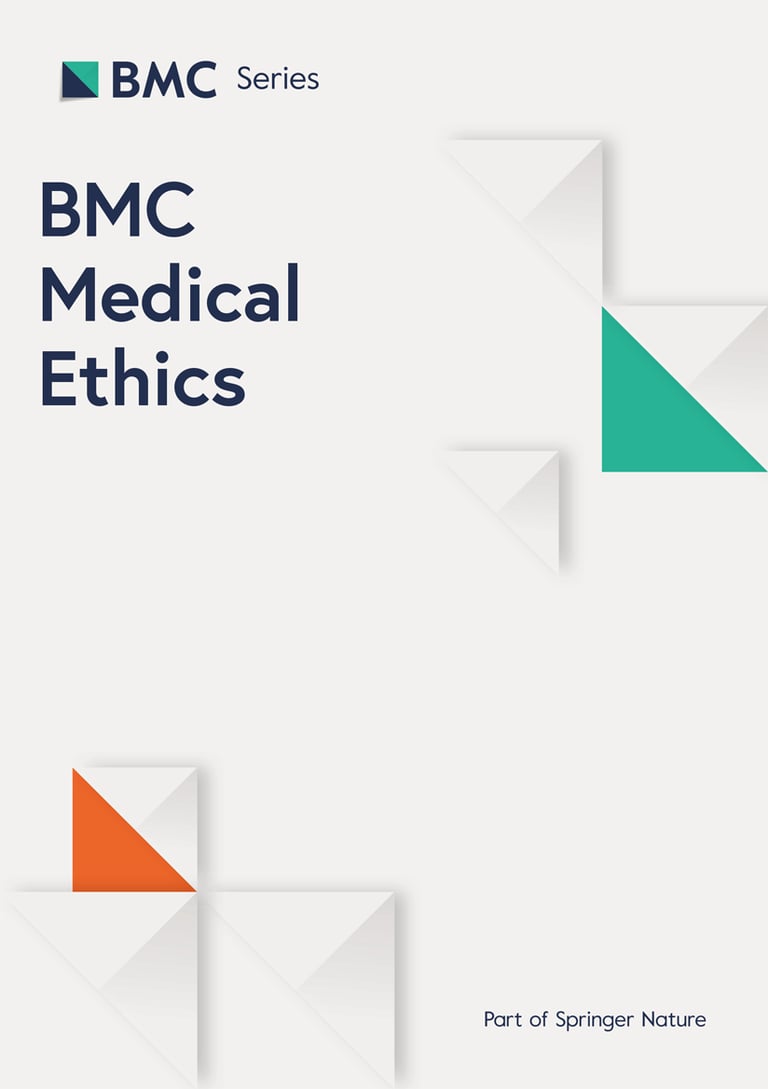
The study underscores the need for robust ethical frameworks, improved AI literacy among healthcare workers, interdisciplinary collaboration, and effective monitoring to address the ethical challenges associated with AI-CDSS implementation.
Emphasizing the importance of interdisciplinary dialogue, the research advocates for the creation of actionable ethical guidelines that accurately reflect the realities of clinical practice.
While participants acknowledged the potential of AI-CDSS to enhance efficiency, they expressed concerns about algorithmic bias, patient autonomy, informed consent, and the impact on the doctor-patient relationship.
From the interviews, five main themes emerged: the tension between efficiency and equity in resource distribution, the necessity for transparency in AI systems, shifting responsibilities in clinical decision-making, ethical issues in data usage, and the challenge of balancing cost-effectiveness with patient-centered care.
Healthcare professionals face complex ethical trade-offs in resource allocation, necessitating a deeper understanding of both the technology and its implications for care delivery.
Artificial Intelligence-driven Clinical Decision Support Systems (AI-CDSS) are increasingly utilized in healthcare for resource allocation, prompting significant ethical concerns that warrant thorough exploration.
A recent study conducted in Turkey involved semi-structured interviews with 23 healthcare professionals to gain insights into their perspectives on the ethics surrounding AI-CDSS in resource allocation.
Additionally, the research highlights a significant gap in existing literature regarding healthcare professionals' views on the ethics of AI-CDSS in resource allocation, indicating a pressing need for further empirical studies in this area.
Participants also recognized the potential of AI-CDSS to improve efficiency but were concerned about exacerbating healthcare disparities and stressed the need for interpretable AI models.
Summary based on 1 source