Argonne National Lab Unveils AI-Driven Protein Design Framework, MProt-DPO, with Exaflop Supercomputing Power
November 8, 2024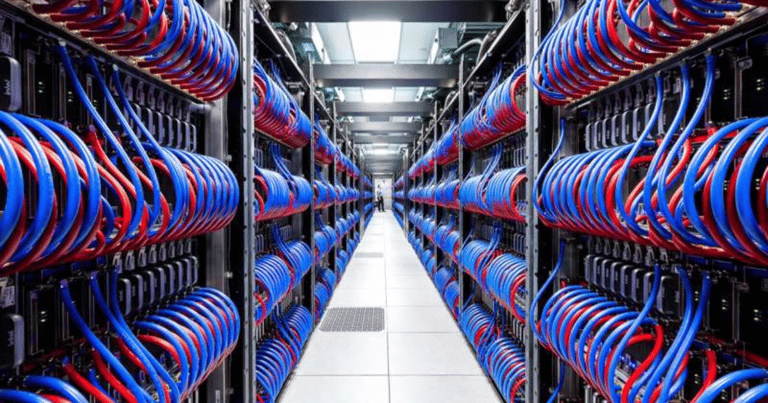
A research team from the U.S. Department of Energy's Argonne National Laboratory has unveiled the MProt-DPO framework, designed to enhance protein design through the use of artificial intelligence and supercomputers.
This innovative framework builds on previous advancements in text-guided protein design by incorporating multimodal data, which significantly boosts the model's trustworthiness and overall performance.
At the core of MProt-DPO are large language models (LLMs), similar to those used in AI tools like ChatGPT, which allow researchers to analyze vast datasets and tackle complex protein design challenges.
One of the major hurdles in protein design is mapping a protein's amino acid sequence to its structure and function, a task complicated by the immense number of possible combinations, as proteins can consist of hundreds to thousands of amino acids.
The framework was successfully tested on the yeast protein HIS7 and the enzyme malate dehydrogenase, demonstrating improved design and efficiency through the integration of experimental and simulation data.
The research team achieved over one exaflop of sustained performance across several supercomputers, with the Aurora system reaching a peak performance of 5.57 exaflops, highlighting the computational power essential for this work.
Arvind Ramanathan, a computational biologist at Argonne, emphasized the framework's potential to discover promising proteins for critical applications, including vaccine development and the design of environmentally friendly enzymes.
MProt-DPO is a key component of Argonne's AI for science initiatives and contributes to the development of AuroraGPT, a model aimed at enabling autonomous scientific exploration.
What sets MProt-DPO apart is its unique integration of multimodal data, which combines protein sequences with experimental results and molecular simulations to accelerate the discovery of new proteins.
The 'DPO' in MProt-DPO stands for Direct Preference Optimization, a method that allows AI models to learn from feedback during the protein design process.
Training the LLMs that power this framework necessitated the use of advanced supercomputers, including the Aurora exascale system located at the Argonne Leadership Computing Facility.
The language models employed in MProt-DPO contain billions of parameters, making the use of supercomputers essential for both training these models and running simulations to verify protein stability and activity.
Summary based on 1 source
Get a daily email with more AI stories
Source
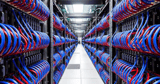
INDIAai • Nov 8, 2024
Researchers leverage AI to speed up protein design process