Revolutionizing Cancer Treatment: New Study Benchmarks AI Methods for Predicting Life-Saving Gene Interactions
October 20, 2024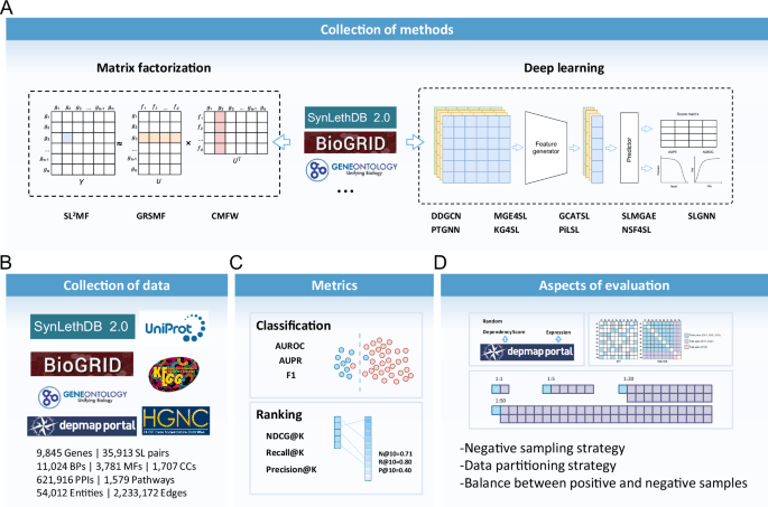
The concept of synthetic lethal (SL) interaction was first identified nearly a century ago in Drosophila Melanogaster, where mutations in two genes lead to cell death, while mutations in either gene alone do not.
This study emphasizes the need for accurate methodologies to predict SL interactions, which are crucial for advancing cancer treatment strategies.
SL interactions have significant potential applications in cancer therapy, as identifying SL partners can selectively kill cancer cells while sparing normal cells.
PARP inhibitors (PARPi) are the first clinically approved drugs that exploit SL interactions, showing particular effectiveness for tumors with BRCA1/2 mutations.
Clinical trials have demonstrated the promise of PARPi for treating various cancers, including lung, ovarian, breast, and prostate cancers, although few SL-based drugs have successfully passed clinical trials due to the challenges in identifying relevant SL gene pairs.
Traditionally, biologists have relied on statistical methods for SL prediction, with techniques like random forests being favored for their interpretability.
While traditional methods for identifying SL gene pairs include wet-lab experimental techniques such as drug screening and CRISPR/Cas9 screening, these approaches are impractical given the vast number of potential gene combinations.
Deep learning methods, which can capture complex patterns, remain underutilized in biology due to concerns over their predictive accuracy and the inherent black-box nature of these models.
A variety of traditional machine learning and deep learning methods were evaluated, including matrix factorization and graph neural network methods, to enhance SL prediction.
The study benchmarks various machine learning methods for SL prediction, assessing their performance across different classification and ranking tasks, while also investigating the impact of negative sample quality.
Different negative sampling strategies were evaluated, revealing that negative samples based on gene expression correlation significantly improved model performance compared to random sampling.
The research highlights the importance of integrating multiple data sources for better predictive performance and underscores the necessity of high-quality training data.
Summary based on 1 source
Get a daily email with more AI stories
Source
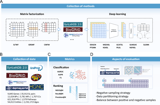
Nature • Oct 20, 2024
Benchmarking machine learning methods for synthetic lethality prediction in cancer